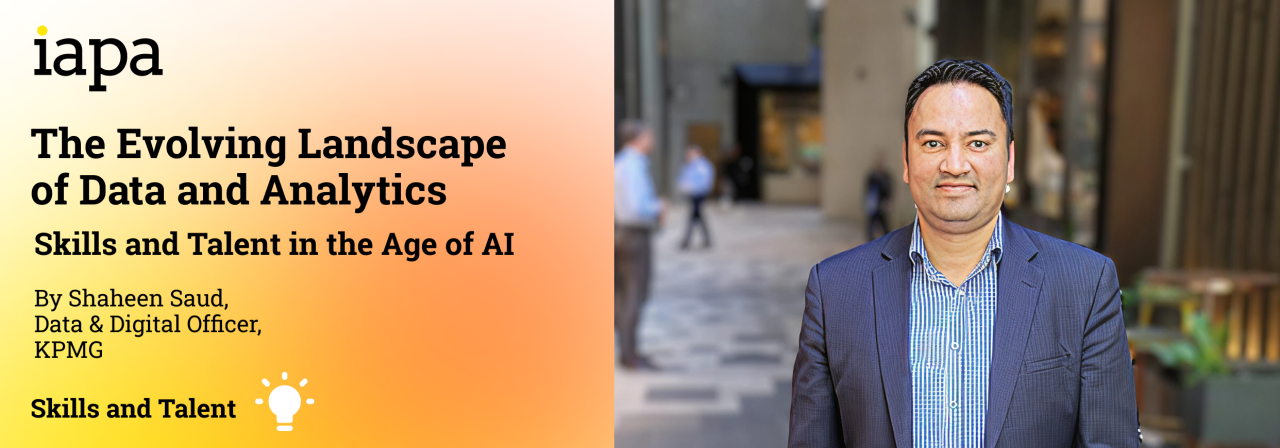
The Evolving Landscape of Data and Analytics: Skills and Talent in the Age of AI
By Shaheen Saud, Data & Digital Officer, KPMG
The data and analytics landscape is evolving at remarkable speed, yet an old adage remains relevant: “the more things change, the more they stay the same”. As technology evolves and AI redefines what is possible, the core objectives remain unchanged navigating complexity, generating actionable insights, and empowering smarter, faster decision-making.
As a technology executive at the intersection of data and AI, I continue to observe how these domains are not only converging but also redefining business strategy, value creation, and the skills required for success. While the tools and platforms are advancing at unprecedented speed, one thing remains clear: success still depends on the human element - the skills, judgment, and creativity that professionals bring to the table.
Organisations are increasingly leveraging AI to transform their business models, products, and services, and AI-ready data is emerging as a critical enabler of that transformation. At the same time, AI is enhancing every stage of the analytics value chain, from data cataloguing and lineage tracking to transformation, visualisation, and insight generation. These advancements are evolving and expanding the role of analytics professionals in driving meaningful outcomes.
Beyond AI itself, the skills and talent needed in analytics are also shaped by broader organisational factors: strategic priorities, the structure of analytics operating models, and the maturity of data and digital capabilities. These elements collectively determine how effectively an organisation can unlock the full potential of analytics and how its people can grow and lead within it.
The Foundation: Core Competencies Remain Essential
1. Data literacy and effective storytelling and communication
Data literacy, the ability to interpret, manipulate, and communicate data effectively is an essential competency for professionals across all sectors. Traditional analytics has long required an analytical mindset and the ability to translate findings into meaningful insights for stakeholders. In the era of AI, this need is even more pronounced, as model outputs can often be complex and opaque to decision-makers. Clear communication of insights, whether through data visualisations, dashboards, or narrative storytelling is critical. While AI can generate analysis, analytics professionals remain indispensable for contextualising results, articulating their implications, and driving actionable outcomes. This reinforces the importance of the "human in the loop" model in AI-augmented organisations.
2. Familiarity with broader data value chain and data capabilities
While an organisation's analytics operating model defines the specific roles and responsibilities within analytics teams, a comprehensive understanding and appreciation of capabilities across the entire data value chain empowers professionals to deliver stronger outcomes and shape their career paths more effectively.
3. Strategic thinking, collaboration and change management
Amid rapid technological advancement, the ability to design innovative strategies to deploy emerging data and analytics technologies has become increasingly critical. Central to this is the need to build cross-functional bridges and foster sustained collaboration across organisations. Equally important is a strong focus on change management, ensuring that new analytics solutions are adopted effectively and sustainably - an area of growing importance for analytics professionals.
4. Statistical thinking
Traditional statistics continues to play a crucial role. AI models are only as good as the data they are trained on and the statistical assumptions behind them. Understanding distributions, variance, hypothesis testing, and correlation vs. causation remains key. This knowledge allows Analytics professionals to interpret results critically, ensuring that insights are both accurate and meaningful.
5. Technical skills
Proficiency in traditional query languages and programming languages used in data analysis is essential. Additionally, expertise in big data platforms as well as knowledge of data pipelines, APIs, and cloud platforms is required for managing extensive datasets, particularly as AI increases the complexity of data pipelines. Data wrangling - including processes such as cleaning, joining and transforming data is a time consuming but critical task. Despite advancements in AI that automate certain activities, analytics professionals must understand data manipulation logic to ensure result accuracy and maintain data integrity.
6. Domain expertise
A deep understanding of the business or domain context cannot be overstated. AI models are agnostic - they do not inherently understand the nuances of a particular industry. Analytics professionals bridge the gap between model output and actionable business insight, contextualising results in a way that aligns with organisational goals.
7. Data management and governance
Analytics professionals must comprehend the available data, the data flow through the value chain, including access, transformations, data privacy considerations, algorithmic bias, and transparency. Evaluating the societal and organisational impacts of AI driven decisions has become a crucial skill in today's environment.
The Shift: New Skills for an AI-Augmented Analytics Future
1. AI fluency
In the age of artificial intelligence, it is crucial for analytics professionals to be familiar with machine learning concepts such as classification, regression, clustering, and neural networks. While deep technical expertise may not be mandatory for every role, the ability to collaborate effectively with data scientists and understand algorithmic results is essential.
2. Prompt engineering
With the increasing prevalence of generative AI and large language models (LLMs), prompt engineering has become a critical skill. Developing precise prompts is essential for obtaining accurate responses. Analysts must formulate appropriate questions to receive summaries, code snippets, visualisations, or model recommendations.
3. Ethics and responsible AI
As artificial intelligence becomes increasingly integrated into analytics, ethical considerations such as data privacy, model bias, and fairness are of paramount importance. It is imperative for analysts to ensure that insights are derived in an equitable and justifiable manner.
4. Agility and continuous learning
AI and analytics tools evolve rapidly, necessitating analytics professionals be agile learners, continuously adapting to new tools and navigating the ever-changing technological landscape. Enhancing expertise requires obtaining certifications, completing online courses, and engaging in practical, hands-on experience.
5. Value realisation
The continuing investment in modern data and AI technology necessitates ongoing monitoring of benefits realisation to help justify the investment as well as provide the case for further investment. Analytics professionals are in a strong position to deliver the evidence base to support return on investment conversations.
Building the Talent Pipeline
To develop analytics capabilities in the age of artificial intelligence, organisations must:
- Promote continuous learning: Facilitate upskilling in AI, coding, and ethics through comprehensive training programs and certifications.
- Encourage cross-functional teams: Integrate data scientists with domain experts, engineers, and business leaders to enhance knowledge transfer across the data value chain.
- Recruit with a hybrid mindset: Seek talent that combines technical skills with creativity, critical thinking, and strong communication abilities.
- Foster culture of experimentation: Encouraging analytics professionals to stay curious, take risks, and learn from failures.
- Develop ethical frameworks: Implement guidelines for responsible AI and data usage that analytics professionals can adhere to in their work.
Conclusion
As we stand at the intersection of analytics and artificial intelligence, it is clear that the future belongs to those who are ready to learn, unlearn, and reimagine their place in an increasingly data-driven world. Yes, the age of AI-augmented analytics is here and it brings with it new technologies, new expectations, and new opportunities. However, the core competencies of analytics remain as essential as ever. In many ways, "the more things change, the more they stay the same."
Our challenge and our opportunity is to blend the best of both worlds: leveraging the power of AI while preserving the human insight that makes data truly valuable.
About the Author
Shaheen Saud is a Data and Digital Officer for KPMG. Shaheen was ranked in the Top 10 of the 2021 cohort of Top 25 Leaders in Analytics.