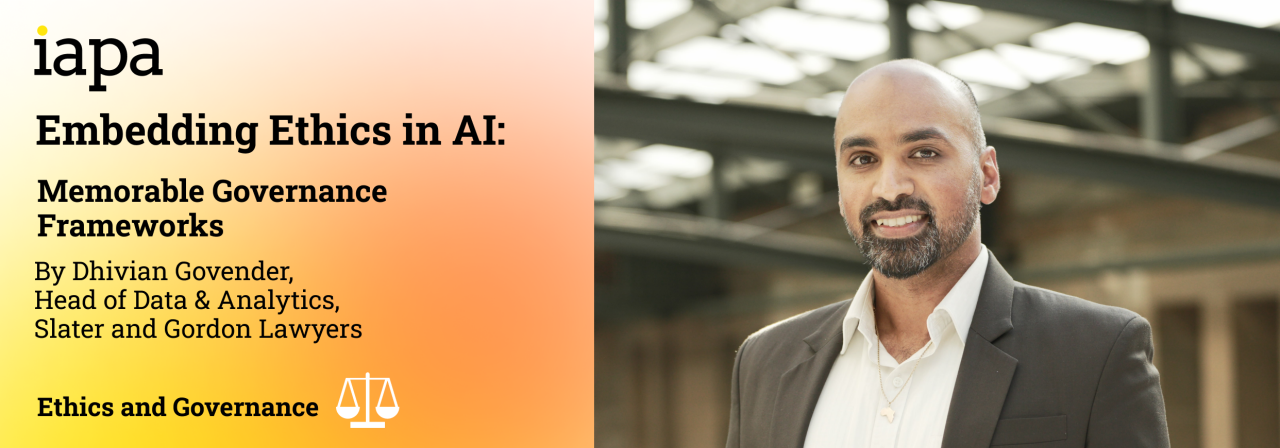
Embedding Ethics in AI: Memorable Governance Frameworks
By Dhivian Govender, Head of Data & Analytics, Slater and Gordon Lawyers
As AI accelerates at breakneck speed, it’s all too easy to get caught up in the momentum and skip over the safeguards - but without strong guardrails and clear governance, the risks can outpace the rewards. Data ethics might not grab headlines, but it's the backbone of responsible AI. That’s why making it resonate - and stick - is more critical than ever.
With AI opportunities popping up in every corner of organisations, the real challenge isn’t spotting use cases - it’s knowing which ones to run with. That’s what inspired me to build a framework for assessing, prioritising, and governing them with clarity and confidence - BEDLAM, a method in the madness. Data scientists can sometimes seem like they’re working magic behind a curtain - brilliant, but baffling to the average observer. In this new era of AI, it’s more important than ever to show the method behind the madness. That’s where clear, practical frameworks come in - to make our reasoning transparent and our decisions understandable.
Let me take you on a quick tour of the six dimensions of the BEDLAM Framework - a way to bring structure to the chaos. Though it was shaped with AI in mind, the thinking behind it can add value to just about any analytics use case prioritisation.
BEDLAM, a method in the madness
- Business benefit: most boards want to see a return on their investment so prioritising based on business benefit is perhaps the most crucial dimension for prioritisation. Use cases that offer the highest business value, allow an opportunity to demonstrate improvements in efficiency, productivity, or profitability. It’s a useful method to build stakeholder confidence and gain support for future initiatives. A formula that has worked for me in the past is: “we will improve business metric X by Y% within Z months/years”.
- Ethics and Risk considerations: placing this dimension directly after the business benefit shows how essential it is. It is pivotal that use cases align to the ethical posture of the organisation. Whilst values and norms can differ across sectors and individual organisations, there are principles which are universally applicable. In Australia, we have the government’s voluntary AI safety standards, which outline 10 guardrails for safe and reliable AI systems. The EU Artificial Intelligence Act also outlines prohibited AI systems and high-risk AI systems. These frameworks and standards provide a great starting point if you’re early on in your AI journey.
- Data availability and reliability: The phrase "garbage in, garbage out" was coined by George Fuechsel, an IBM programmer and instructor, in the early 1960s. Today, data is often referred to as the fuel for AI because it powers the algorithms. Quality data enables AI to learn effectively, uncover meaningful patterns, and allows for informed decision-making. Poor-quality data can lead to biased, inaccurate, or misleading results, undermining the value and trustworthiness of AI applications. Therefore, ensuring data availability and reliability is essential for harnessing the full potential of AI and achieving successful outcomes. A way to speed this up is by having data that is well catalogued with business metrics that are well-defined.
- Longer term application/benefits: a general principle with regards to innovation is creating something that has multiple applications. An AI solution with broad application across an organisation is more beneficial than one with a very niche application. When prioritising use cases, including this dimension means that the assessment exercise factors in the application beyond the prototype/pilot that is initially identified.
- Alignment to strategy: Most modern organisations either have a current transformation strategy in place or are going through a refresh of their existing strategy. It is essential to align AI use cases with those strategic goals of the organisation. Successful AI implementation generally allows for better insights, reduction of cost and enhanced operational efficiency. One of these factors is typically a fundamental part of an organisation’s strategic goals. Therefore, aligning AI use cases with these strategic outcomes ensures that the prioritisation of AI initiatives is set up for success. An in-depth understanding of the overall transformation or strategy refresh is essential.
- Magnitude of input: there are several factors to consider within this dimension. The costs of the AI solution are one factor (setup costs, license fees and ongoing compute costs). A factor that is often overlooked is the integration with the existing ecosystem and infrastructure of the organisation. With prototyping generally existing in low-tech constructs, this can be understated if the solution would require specialist, skilled personnel to maintain, introducing “tech debt”. In my opinion, the most important factor in this dimension is the change management effort required. Stakeholder education and end-user training is likely required, whenever there are new interfaces introduced. Additionally, incorporating domain expert input when assessing use cases provides a more comprehensive perspective on this dimension.
Sure, there are plenty of other layers you could add - but in my view, these are the essentials. When it came to naming the framework, I toyed with a few acronyms, but BEDLAM stuck because it’s easy to remember. Let’s face it: in the world of data and AI, topics like ethics and governance can come off as dry or hard to engage with. That’s why, as analytics professionals, we’ve got to make our ideas resonate and stick - not just technically sound, but truly unforgettable.
About the Author
Dhivian Govender is the Head of Data and Analytics, Slater and Gordon Lawyers. Dhivian was also ranked #2 in the 2023 cohort of Top 25 Leaders in Analytics.