Effective machine learning starts with considered human thinking
09 Oct 2017
By: Brad Howarth, researcher, speaker and author
Innovation makes a regular habit of outpacing those responsible for regulating its usage.
Unfortunately for the regulators, that pace is quickening. In the current era of agile development a mantra exists that says getting a product or feature to market as quickly as possible is the best strategy, regardless of its flaws, as the market will soon determine whether it succeeds or fails.
But the unfettered advancement of technology can quickly lead to consequences that are unforeseen – and unfortunate.
Already we have heard luminaries such as Elon Musk and Google DeepMind’s Mustafa Suleyman petition US regulators to ban lethal autonomous weapons – the so-called killer robots.
But while at first blush their fears might seem fanciful, it is also worth considering that the technology they describe is not resident in some dystopian future, but is possible today.
The realm of data science is not immune to unforeseen consequences, leading many to question what safeguards exist as we push further ahead with technologies such as analytics and AI.
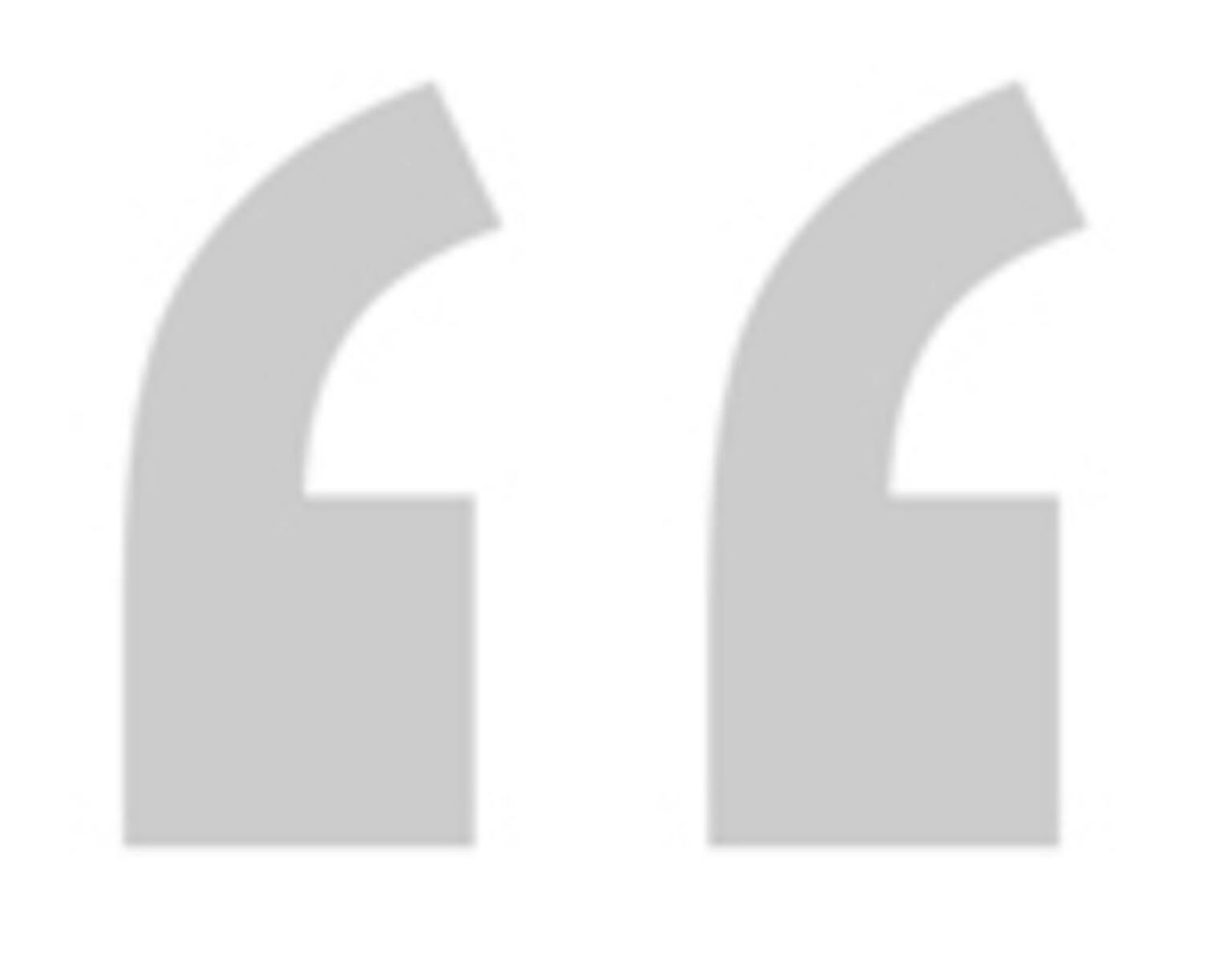
It is like we have built a hot air balloon, and now we are in the air, asking how do we steer.
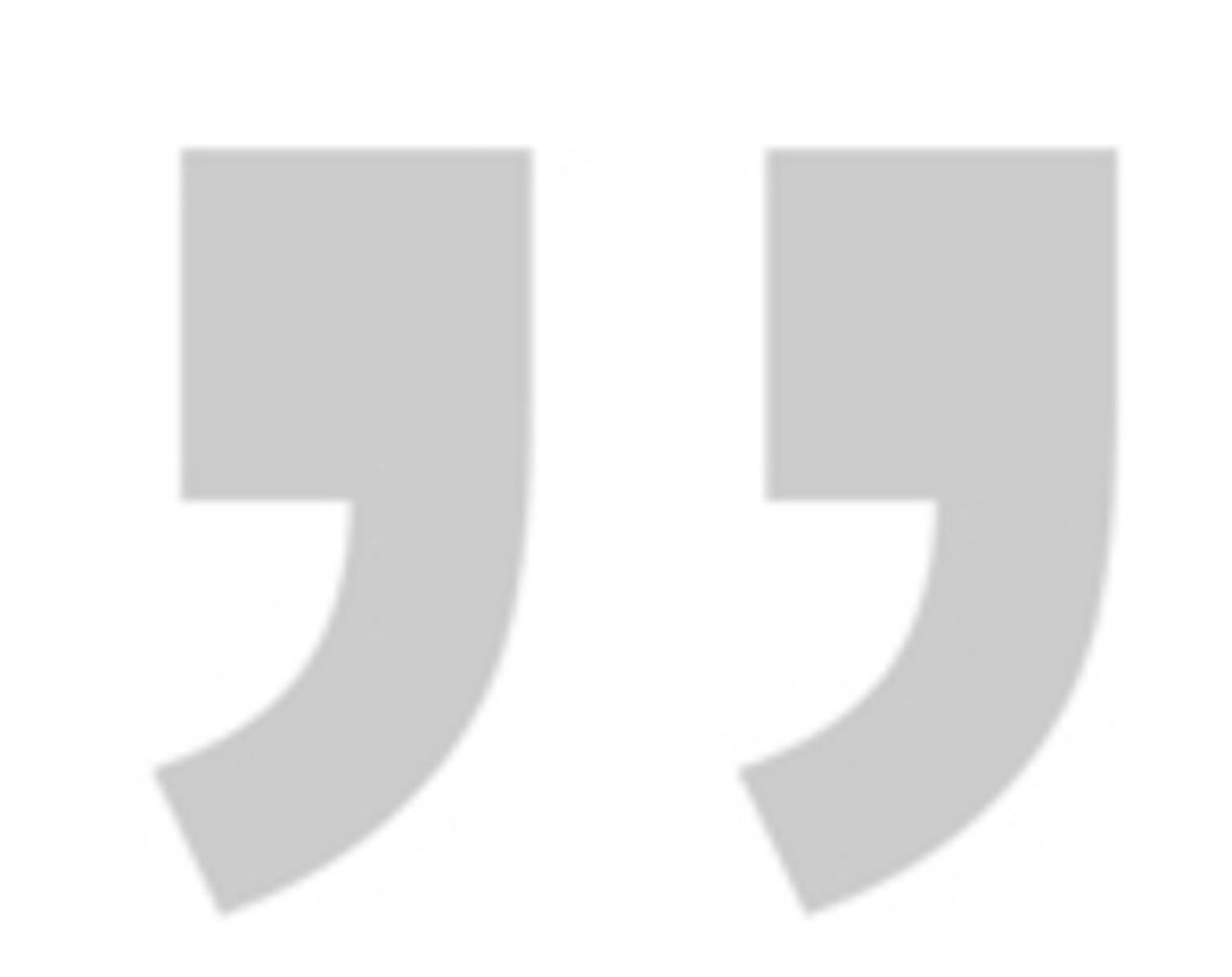
It is a topic that has been on the mind of Halim Abbas, a data scientist who has worked in fields including ecommerce and healthcare. He is currently the head of data science at Cognoa, which is building AI-powered cognitive screening tools that look for developmental delays in young children. Abbas will be exploring how predictive analytics can transform health and change lives at the IAPA 2017 national conference Advancing Analytics, being held in Melbourne on October 18.
“I am not satisfied with the current status of the ethical frameworks surrounding AI,” says Abbas. “It is something that has been overlooked for too long, and now we are trying to catch-up. We have built something, and it is turning out to be a very powerful driver in society, and we are not really sure how to best use it. It is like we have built a hot air balloon, and now we are in the air, asking how do we steer.”
One of the greatest concerns for Abbas is data privacy, thanks to AI’s ability to connect separate pieces of data to build profiles of people, and then make decisions about them.
“It is very hard to govern what sorts of data can and cannot be used by AI, and in what ways, to effect an understanding of a person that could constitute a breach of privacy,” Abbas says.
Having worked in both healthcare and ecommerce, Abbas has seen how the ethics of data usage can vary greatly from industry to industry.
“For example, when Netflix does some work to improve the recommendation of a movie to a user, there isn’t a big standard of evidence that applies before you actually deploy it,” he says. “If there is an inclination it might work, then good, just ship it.
“But when it comes to healthcare, the process is much more rigorous, probably to the extreme.”
Hence while a consumer might be prepared to let the machines do the thinking with no chance of interrogation – a so-called ‘black box’ scenario – a clinician will want to know how the conclusion was reached. Hence at Abbas’ own company, all developments are validated through blinded clinical experiments.
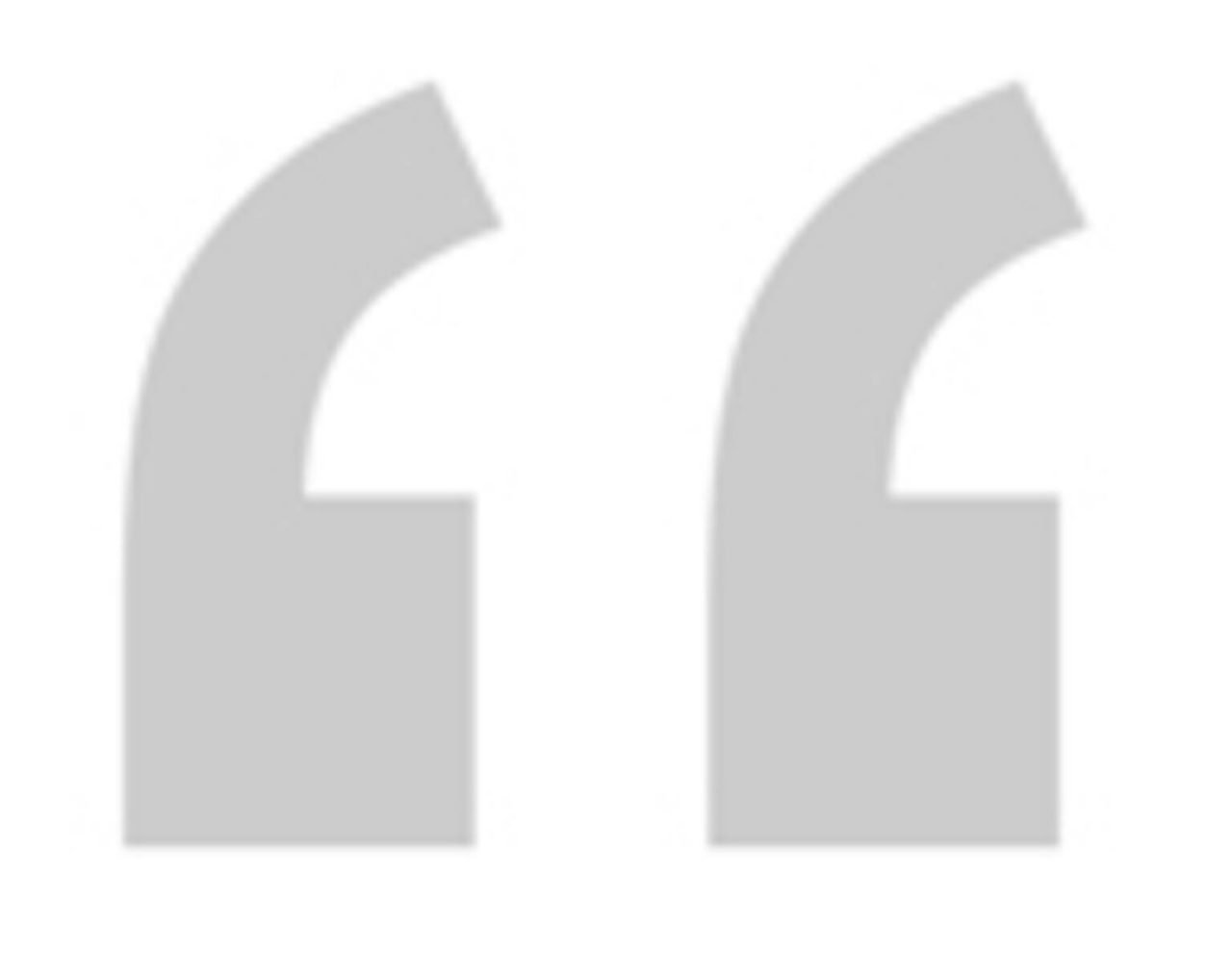
There is wide open space for AI viruses to fool these machines, and no one seems to be worried about building virus-proof systems
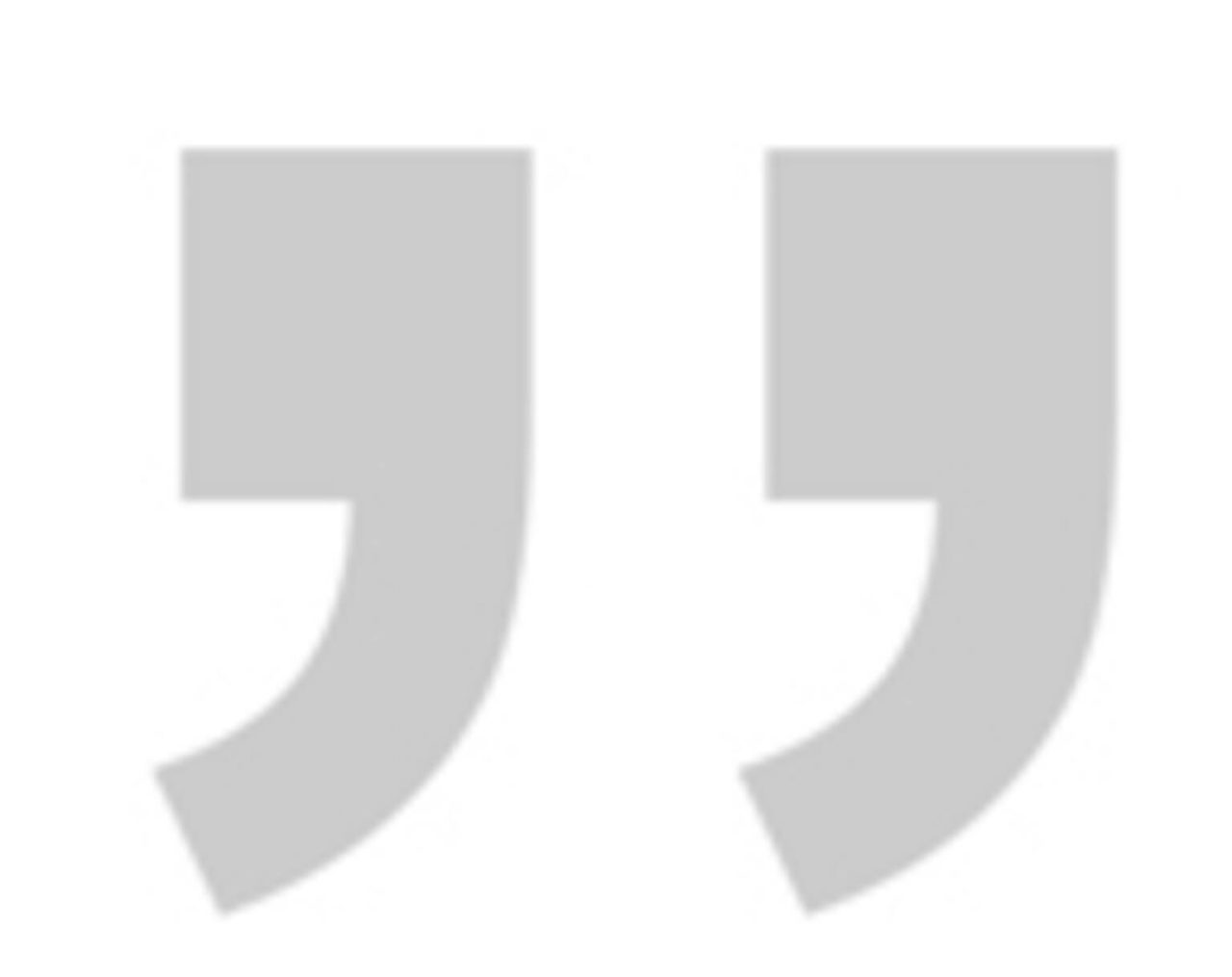
According to the data scientist, investor, consultant and former chief data officer for the City of Chicago, Brett Goldstein, many of the unintended consequences of data science can arise from the tools being used by people who haven’t received appropriate training.
“We have created a series of powerful tools, but these tools can give you very invalid answers, and if you don’t understand how they work, you can go down the path of flawed decisions,” Goldstein says. “There needs to be a real focus on making sure people understand what the error could be, and the difference between correlation and causation, as well as the difference between classical and machine learning techniques. Because at the end of the day there are remarkably few cases where data will give you the absolute perfect answer.”
He says the problem is exacerbated by the fact that people don’t usually create flawless software.
“We still have bugs, we still have problems,” Goldstein says. “So what happens when you write a complex algorithm and there are a couple of lines of bad code, and those create unforeseen conditions? That is pretty problematic, especially as these algorithms increasingly impact people’s livelihoods.”
Goldstein has sought to alleviate this problem by working in conjunction with the University of Chicago to create a new graduate degree program, the Master of Science in Computational Analysis and Public Policy. Goldstein will also be talking further about how data and analytics can transform a city at the IAPA national conference “Advancing Analytics”.
Like Abbas, he advocates against the idea of black box systems in favour of transparency, and urges both service users and creators to rally against them. He is embedding this thinking in the start-up he has co-founded, a predictive-policing company called CivicScape, which is releasing all of its own code online through GitHub. The hope is this will improve performance and help engender trust from law enforcement and local communities.
“You should be nervous of algorithms that don’t show their code, because you should be able to check the underlying math,” Goldstein says. “Our belief with CivicScape is that you can still create a good business and post your algorithms, because the more eyes the better. As you improve your algorithms, you get the public’s trust, so transparency can be a win-win for both sides.”
Abbas says other unintended outcomes arise as different systems are linked together. For instance, it is now possible to unlock a door remotely over the network, which led some developers to link that functionality to Amazon’s Alexa home AI solution. He suggests thieves need only walk along streets shouting ‘Alexa, unlock the front door!’
“As AI systems become more prevalent in everyday life hackers will be thinking of ways to hack the system,” Abbas says. “Right now with AI we are where we used to be in computing 30 years ago, when people hadn’t yet developed computer viruses. Everything was vulnerable because there was no such thing as an antivirus.
“There is wide open space for AI viruses to fool these machines, and no one seems to be worried about building virus-proof systems.”